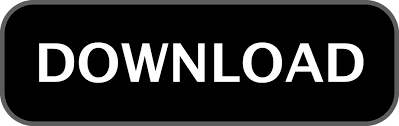
Three classes of EQ modes and two classes of BM modes were found in linear operators, defining a 6-class taxonomy. The equalization mode (EQ) introduced in this work focuses on cluster sizes, while the brightness mapping (BM) mode describes the CIE L* luminance distribution per cluster. To visualize the way operators work the sizes of the clusters and the average brightness of each cluster are plotted. Based on the fact that most operators assign each of the $256^3$ colors a single gray level, ranging from 0 to 255, they are clustering algorithms that distribute the color population into 256 clusters of increasing brightness. In this article, the conversion of color RGB images to grayscale is covered by characterizing the mathematical operators used to project 3 color channels to a single one. Experimental results conveyed the remarkable performance and diversity of our proposed method. Furthermore, the joint intensity–gradient constraint in data-fidelity term is proposed to limit the degree of freedom within generative model at the iterative colorization stage, thus being conducive to edge-preserving colorization effect. As the score function is determined, data samples are generated by means of annealed Langevin dynamics, forming an iterative colorization procedure. Specifically, a set of intensity–gradient formed high-dimensional tensors are trained, via the score matching, to attain the gradient of data distribution in joint intensity–gradient domain. Considering that the number of samples in score-based generative model has influence on estimating the target gradients and the gradient map possesses important latent information of the image, the inference process of the generative modeling is conducted in joint intensity–gradient domain for colorization. Although unsupervised learning methods have shown the capability to generate plausible color, inadequate exploration of detailed information and data dimensions still limit the performance of the colorization model. This paper proposes an iterative score-based generative model for solving the automatic colorization problem. Third, we demonstrate that an effective luminance distribution can be achieved using our algorithm by using global and local tone mapping applications. Second, our optimal color conversion method produces luminance in images that are comparable to other state of the art methods which we quantified using the objective metrics (E-score and C2G-SSIM) and a subjective user study. Our main contribution is threefold: First, we implement a high-speed color processing method using exact pixel by pixel processing, and we report a $5.7\times$ speed up when compared to other new algorithms. In this proposed color to gray conversion model, we implement a weighted blending function to combine red (perceived warm) and blue (perceived cool) channel. This phenomena creates a perception of warm colors "advancing" toward the eye, while the cool colors to be "receding" away. It causes some rays corresponding to cooler colors (like blue, green) to converge before the warmer colors (like red, orange). Chromatic aberration results from differential refraction of light depending on its wavelength. In this paper, we propose a light-weight and high-speed image decolorization method based on human perception of color temperatures. However, some image information is lost when converting from color to grayscale.
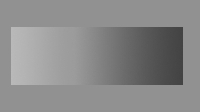
Grayscale images are fundamental to many image processing applications like data compression, feature extraction, printing and tone mapping.
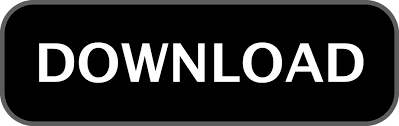